Data annotation, the workhorse behind AI and ML algorithms, creates a highly accurate ground truth that directly impacts algorithmic performance. Annotated data is critical for accurate understanding and detection of input data by AI and ML models.
Contents
- What is data annotation in machine learning?
- How to annotate data in machine learning?
- Data Annotation Challenges for AI & ML companies
- Key advantages of employing data annotation for AI and ML models
- 4 major types of data annotation and labeling
- Future of data annotation with technological advancements
- Conclusion
Smart equipment and smart life have become an integral part of our daily life. Right from self-driving cars, smart and nudge replies to emails, estimating time of arrival through GPS app to next song in the streaming que – everything is powered by Artificial Intelligence (AI) and Machine Learning (ML).
If market experts are to be believed, AI has the potential to deliver additional global economic activity of around $13 trillion by 2030.
– McKinseyTo do all these, AI and ML models are to be fed with training data; a lot of data. Computers can’t process visual information the way human brains do. A computer needs to be told what it’s interpreting and provided context in order to make decisions. Data annotation for machine learning makes those connections.
What is data annotation in machine learning?
Data annotation provides context and meaning to raw data, enabling machine learning models to recognize patterns, make predictions, and perform complex tasks. Annotated or labeled datasets ensure that training models accurately interpret and classify new, unseen data; like humans do.
How to annotate data in machine learning?
Data annotation process in machine learning involves labeling datasets to provide context and meaning, enabling algorithms to recognize patterns and make accurate predictions. The process starts with gathering raw data, such as images, text, or audio. Annotators then tag or label this data with relevant information, such as bounding boxes for object detection in images or sentiment tags in text.
In absence of human annotated datasets, AI and ML algorithms cannot compute the essential attributes with accuracy and ease.
Data Annotation Challenges for AI & ML companies
Applications of artificial and machine learning platforms are becoming commonplace. However, a thick layer of hype and fuzzy jargons shadow the challenges AI and ML companies face in terms of feeding accurately annotated training datasets.
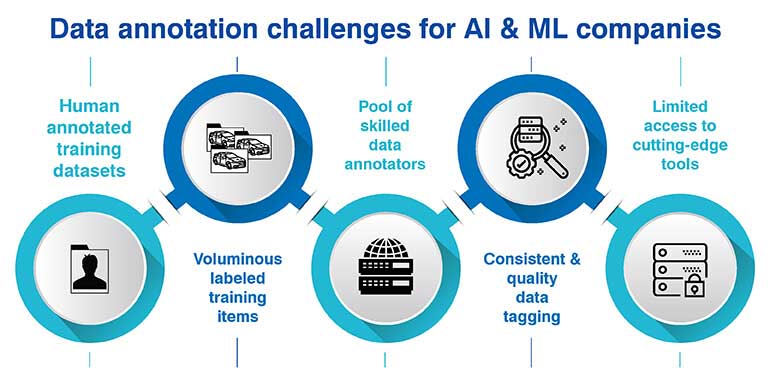
Higher quality training datasets: The quality of annotated data decides the fate of AI and ML project. To train a model to recognize patterns and relationships between variables; AI and ML companies have to feed accurately annotated datasets. Analytics companies cannot afford misaligned bounding boxes and confusion in the classifiers. These mistakes can prove to be disastrous. Not to forget, the ability of AI and ML models to deliver personalization and efficiency depends on precisely curated and labeled data.
AI and ML models are data hungry: ML projects typically require thousands or even millions of labeled training items to be successful. While the goals of machine learning projects can vary widely in complexity, they all share a common requirement: a large volume of high-quality data to train the model.
According to McKinsey Global Institute 75% of AI and ML projects demand learning datasets to be refreshed once every month, and 24% of AI and ML models require a daily refresh of annotated datasets.
Resources for data annotation projects: AI and ML companies don’t have adequate manpower to handle large-scale and complex annotation projects. Pulling in engineers or other team members off their core tasks to perform data labeling tasks proves expensive. In absence of progressive flow and accurately annotated data; AI and ML companies cannot develop models capable to rightly interpret important attributes or make accurate predictions.
No wonder the global data annotation market is about to skyrocket from US $695.5 million in 2019 to US $6,450.0 million by 2027.
Elevate Your AI with Data Annotation
Click to Begin »Key advantages of employing data annotation for AI and ML models
Text annotation, image annotation or video annotation facilitates a deeper understanding of the meanings of the text or objects, thereby allowing algorithms to perform better.
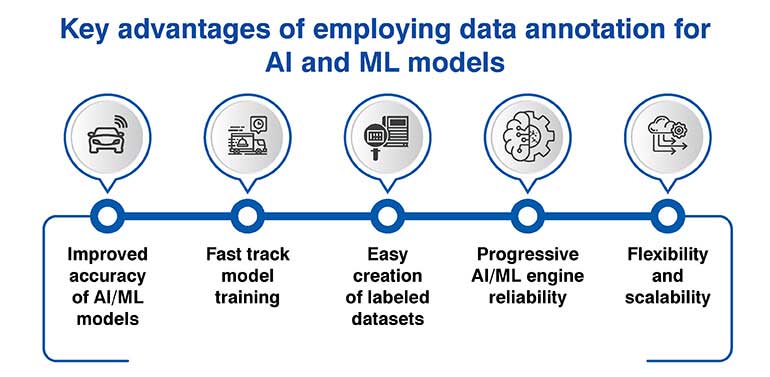
- Improved precision of AI and ML models
A computer vision model operates with different levels of accuracy over an image in which several objects are labeled accurately as against an image in which objects haven’t been labeled or poorly labeled. So, better the annotation, higher is the precision of the model.
- Fast track model training
Machine learning project TAT reduced by 54% for a data analysis services provider. Data annotation company studied the footage of a traffic signal to identify and label vehicles by their category, model name, color, and the direction it is traveling into. It is only through this accurately annotated database that an AI & ML model understands what it needs to do with the data being fed to it. The model, thus, fast learns to apply the valid treatment(s) to the labeled data and generates results that make sense.
- Easy creation of labeled datasets
Annotation of any form of data streamlines preprocessing, which is an important step in the machine learning dataset building process. In a classic case, 40,000+ images were labeled and fed into machine learning models, using a blend of manual and automated workflows. It helped a Swiss data analysis solutions company resolve the issue of food wastage for leading hotels and restaurants. Regularizing data annotation services, as a result, leads to the creation of massive labeled datasets over which AI & ML models can functionally operate.
- Streamlined end-user experience
Well-annotated data offers altogether a seamless experience to the users of AI systems. An efficacious intelligent product addresses the problems and doubts of users by providing relevant assistance. The capability to act with relevance is developed through annotation.
- Progressive AI engine reliability enhancement
The axiom that increasing data volume increases AI model’s accuracy and precision hold true only when there is a perfect data annotation process to supplement the models with labeled data. So, as the data volumes soar, the reliability of AI engines, too, increases.
- Imparts ability to scale implementation
Annotated data can easily accommodate sentiments, intents, and actions from multiple requests. It also facilitates the creation of accurate training datasets, thereby imparting AI engineers and data scientists the ability to scale the mathematical models for diverse datasets of any volume.
4 major types of data annotation and labeling
Data annotation for machine learning is a broad practice, but every type of data has a labeling process associated with it. Some of the commonly used annotation types include:
1. Text annotation
Text annotation is common in search engines, where words are tagged to enable search engine algorithms to load the pages containing the search keywords. Tagging helps in matching the keywords with URLs in the databases and allows search engines to fast produce desired results for searchers. Here is a practical insight:
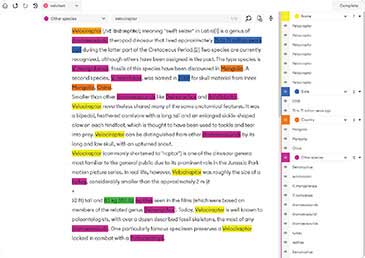
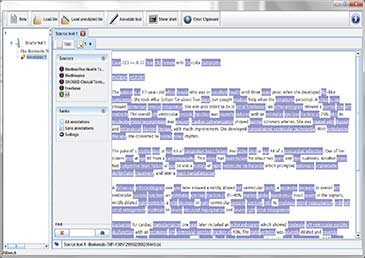
2. Video annotations
Amongst many use cases, an autonomous vehicle is the one in which video annotation proves vital. Technically, it divides a video into frames, and each of them categorically identifies the object(s) of interest. As a result, video annotations offer tremendous visibility into the road traffic pattern, in-cabin driver actions, accident-prone spots, etc., and thereby significantly boost on-road safety.
A California based data analytics company hired a data annotation company to label pre-recorded and live video streams to power their machine learning models. It helped them successfully deploy a dashboard of directional traffic volumes that provided live data and alerts based on historical volumes for city traffic management.

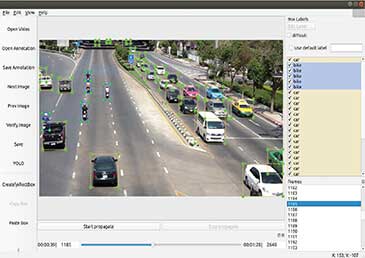
3. Image annotations
200 million accurately annotated images empowered the world’s leading technology company to enhance search engine experiences for its clients in the U.S. and international markets. A highly accurate training dataset enabled users to find images on time; that is free of spam and relevant to the search query intent.
Applied using a range of techniques such as bounding boxes, polygons, tracking to masking, image annotation involves labeling objects of interest in an image. Elements are pre-determined by machine learning experts to supplement the computer vision models with the requisite knowledge. As decided by the context, a combination of techniques can be used to label objects in an image
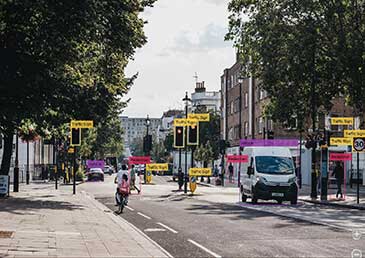

4. NLP annotation for speech recognition
Transposing complex grammar rules into 14 languages, pronunciation checks, validated transcription was used to train a virtual assistant to better understand and respond to queries of 150 million+ active users per month.
In NLP annotation, the language is the focus, and tagging is used to unravel the deepest insights from the nature of the language. The NLP annotation process comprising of Parts of Speech (POS) Tagging, Phonetic Annotation, Semantic Annotation, Key phrase Tagging, Discourse Annotation, etc. capture properties of linguistic structure. It empowers ML systems to interpret meanings and understand contexts as humans do.
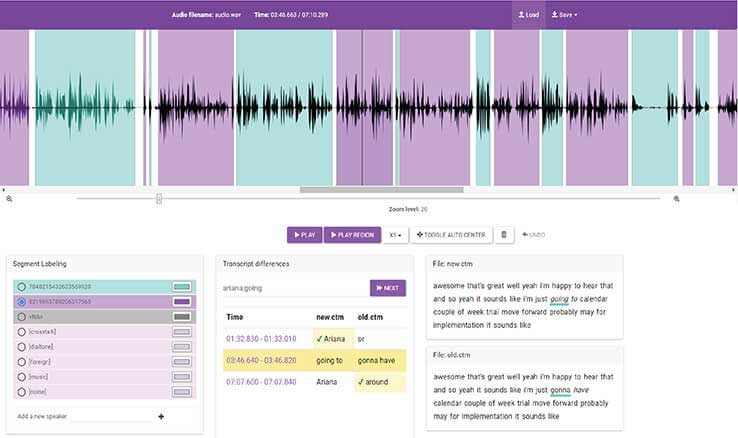
Future of data annotation with technological advancements
A study by Grand View Research has noted that the global data annotation market will reach 1.6 US billion dollars by 2025 and Research & Market’s report projects the global data annotation market at 6.45 US billion dollars by 2027.
Altogether, a massive positive forecast for the data annotation market can be attributed to following future technological trends in space.
- Smart labeling tools will dominate the future AI and ML landscape. Backed with predictive analytics, data labelling capabilities will be fully automatic, detecting labels without any manual intervention.
- Reporting frameworks will be an integral component of data annotation processes. Operational intelligence will offer an understanding of how annotation complexities are being handled. The reporting capabilities will be an essential add-on to monitor the annotation throughput and productivity.
- With a need to sustain accuracy levels, automation plus strong quality control is essential to justifiably annotate high-volume data. This will be a key character of next-gen data annotation, where not sheer labeling but gauged and quality labeling will be the true focus.
- Any type of annotation or labelling services are heavily relied upon to improve the performance of machine learning projects. They use a combination of skilled human annotators, annotation tools, and verified workflows to produce, structure, and label high volumes of training and testing data.
At HabileData, we provide leading-edge data annotation services. Our team provides the most precise and complete labeled datasets for your AI projects. Our data annotation services adapt to AI and machine learning projects. We stay current with industry capabilities to provide accurate, high-quality annotations that support your AI efforts. Our precise data labeling helps your AI models perform well and make a difference.
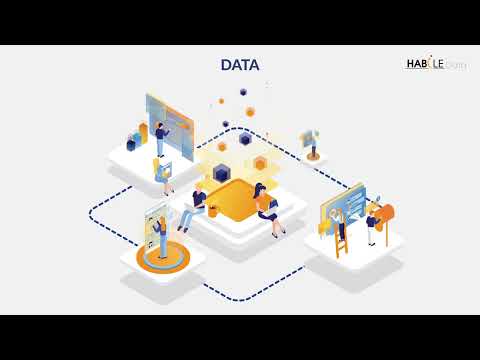
Conclusion
The right application of data annotation is possible only when you leverage the fine combination of human intelligence and smart tools to create high-quality training data sets for machine learning. MIT Technology Review Report rightly says that rightly annotated data has been the biggest challenge to employing AI. Enterprises should build strong data annotation capabilities to support AI & ML model building and prevent it from failing miserably. We, humans, are a notch above computers since we can better deal with ambiguity, decipher the intent, and several other factors that impact text, video or image annotation.
Accurately annotated data determines whether you create a high-performing AI/ML model as a solution to a complex business challenge, or you wasted time and resources on a failed experiment. And when lacking time and resources to build such capabilities, consulting data annotation companies is a smart move. Apart from time and dollar optimization, data annotation specialists allow you to rapidly scale your AI capabilities and conceptualize machine learning solutions to match the market requirements and meet customer expectations.
Experience the Power of Data Annotation
Start Your Free Trial Today »