Data cleaning always attracts questions about how, why and what’s the best form of B2B data cleansing. Coherent plan backed by technology solutions and executed by data cleansing specialists prove to be force multipliers that arm aggregators with high quality data to make profitable growth.
Maintaining data quality is a serious task for B2B data aggregators, and they spend a significant chunk of their best resources to keep contact list, profile, product, customer, sales, demographics and other data clean, accurate and updated.
According to HBR 47% of newly created data records carry at least one major error that affects work. And we are talking here of the fresh data in databases, not just old records that everyone knows need cleansing and enrichment.
High-quality data gathered from different sources requires validation & makes data cleansing a mandatory and ongoing operation for data aggregators.
Challenges in maintaining B2B database quality
Dirty data is a serious problem for businesses. Poor data quality costs the US economy approximately $3.1 trillion annually. Garbage in, garbage out is what it is. The challenges of B2B data aggregators have also multiplied with the entire digital world backed by data shifting to real-time data streams. Combining datasets from multiple sources is critical when the quality of the output affects business revenues. The only way to address this is to identify erroneous data and take steps to rectify it.
10 common issues with incoming real-time data or data acquired from disparate sources
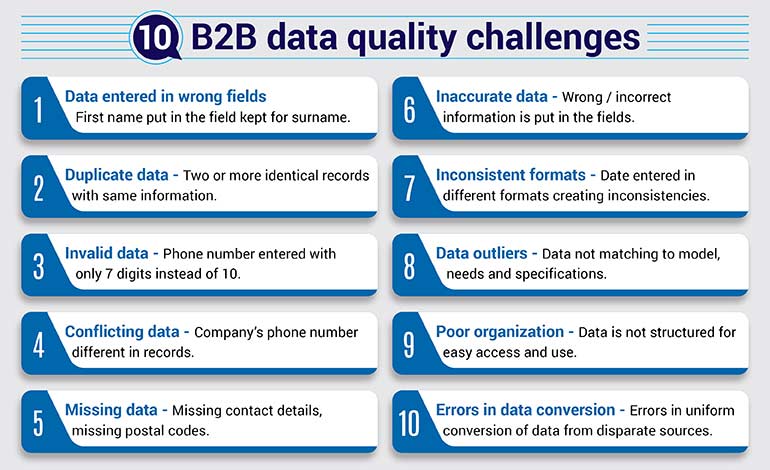
Ultimate B2B data cleansing tips
Dirty data, data errors, data in varied and inconsistent formats and similar challenges are inevitable in data aggregation. Cleaning the data and making it usable for clients requires putting in place B2B data cleansing best practices, before you initiate the data cleansing process.
1. Creating a data quality plan
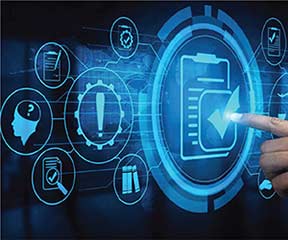
Creating a plan is necessary for a project of any kind and is applicable to data cleansing also. With 27% of data aggregators not sure of how accurate their database is, it is critical to chart out a data quality plan to establish a realistic baseline of data hygiene. Cleansing static databases at backend before it enters the system, cleansing real-time data streams, its source, its destination, etc. are the factors that can make the strategy differ.
Here are tips to create a data cleansing plan to maintain quality of your B2B database:
- Determining and focusing on the model and metrics.
- Creating data quality key performance indicators (KPIs) to track data health.
- Laying out the initial data inspection process.
- Doing quick sample checks to identify problematic datasets.
- Determining the intensity of detailed effort, tools and models to use for cleansing specific datasets.
- Setting up checks to prevent ‘over-cleaning’ of data.
- Beginning data cleansing and error rectification.
- Validating ‘clean data’ and generating reports.
- Sending data to the database after thorough quality clearance.
- Checking the overall quality of data by a plausibility analysis and by comparing new data against previous sets. Anomalies in patterns and outliers can as easily arise from errors, as from genuine changes.
2. Managing data entry with RPA and AI-based technologies
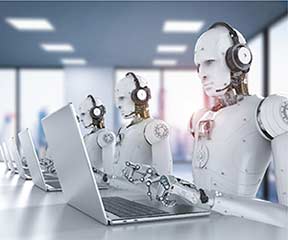
Checking the problem at source, before it gets entry to the aggregator database is the first critical step to take. 309,000 records entered within 45 days to deliver a robust, drilled down and easy to access database to California bar council with help of technology driven data entry. The shift to real-time data has also made automated data cleansing essential. Except in cases of outliers, where considered decision needs to be taken, real-time data entry depends on automated cleansing with the use of RPA, AI and machine learning.
- Checking data at the point of entry to master database ensuring only standardised information passes through.
- Creating a Standard Operating Procedure (SOP) for the entry of quality data.
- Using RPA and AI-based tools for lifting data standardisation accuracy.
- Catching and correcting errors in real-time with automated solutions.
- Parsing formats and ensuring proper merging of data from multiple sources.
- Using automation to revise and update schema to match that required by the master database.
With real-time data, the ideal solution is to have data engineers and data scientists monitor the incoming data streams, detect errors and correct issues with help of automation before the data is sent to the data warehouse. Without proper and automated checks and balances, introducing real-time data into the database, even if the data is pristine in quality, can create lack of synchronization in metrics and inaccuracies in fact tables.
3. Detecting and processing outliers
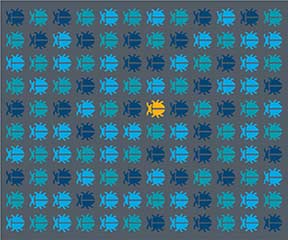
Outliers form a special case in data cleansing and must be tackled with extreme care. Detecting outliers, analysing them and processing them first is required to prepare datasets for machine learning models, and consequent real-time or near-real-time automated data cleansing. Processing outliers and enriching 17 million+ hospitality records drive efficiencies in marketing campaigns for a hospitality data aggregator company.
Outliers are usually detected through data visualization methods and methods like Z-Score (parametric); linear regression, proximity-based models and others.
Data outliers are caused due to:
- Human errors.
- Errors of instruments or systems.
- Data extraction or planning/execution errors.
- Dummy outliers placed to test viability of detection process.
- Errors in data manipulation or unexpected data mutations.
- Errors in mixing data from multiple or wrong sources.
- Genuine changes or novelties in data.
Common steps taken to address data outliers include:
- Thoroughly exploring the data.
- Setting up filters to catch outliers.
- Removing or changing outliers to conform.
- Changing outlier value with tools like winsorized estimators.
- Considering the underlying distribution before deciding the fate of outliers.
- Identifying mild outliers, and segmenting and analysing them thoroughly.
Dealing with outliers is always critical and whether an outlier should be considered an outright anomaly depends upon the model and the analyst, who is qualified to decide what to do with the data point. Extreme values can be removed by data trimming, but the usual practice is to decide whether changing the outlier value to one that matches the dataset will help or hurt cleansing, and then do it.
Hire our experts for advanced B2B data cleansing.
Establish contact with your ideal prospect & turn them into leads.
Talk to our experts today »4. Using classification models to influence merge/purge
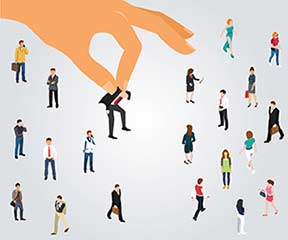
Data comes from multiple sources, and there are chances of duplicates or bad data in any dataset. The data is clustered by the process of merge/purge which stores the data at one place removing redundant records. Often duplicate records also contain unique data, like one may have an email address of the customer while the other may have his cell number. So duplicate data can’t be removed arbitrarily.
- Merge different databases from disparate sources like Excel, SQL server, MySQL, etc. into a common structure.
- Identify duplicates.
- Use advanced data matching tools.
- Remove/purge the data not needed.
Use advanced data matching techniques in merge/purge process, which will ensure that only one record is generated retaining all required information, removing the duplicates. This is especially important when dealing with huge mailing lists or contact/profile lists.
5. Leveraging imputation techniques to address missing values
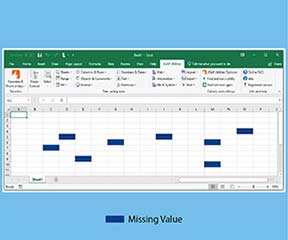
One of the key challenges in data cleansing is handling of missing values that distort analytic findings. Dropping of the columns with missing values is the simplest way to address the issue. Using list-wise deletion and pair-wise deletion is ineffective when working with large datasets. Regression is another way to handle missing data with high computing power.
Current data imputation techniques include:
- Migrate to machine-learning based processes and imputation techniques.
- Exploring and identifying different imputation techniques before treating missing values.
- Using average imputation and common point imputation wherever feasible.
6. Constantly driving data relevance analysis

Relevance analysis is important to turn data into useful and actionable information. Constant data relevance analysis interprets data giving it context.
Relevance analysis includes:
- Building intelligent platforms for visual and numeric insight into data quality metrics.
- Doing away with obsolete data to avoid incurring unnecessary data storage costs.
- Making sure data is actionable.
Read also:
5 step process to clean bad sales data7. Regularizing data cleansing and monitoring of B2B databases
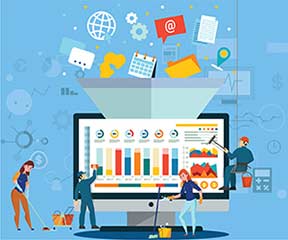
Bad data leads to high bounce, low clicks and even lower conversions. An analysis of over 775,000 leads from B2B marketers done by Integrate, a demand generation software platform showed that 40% of leads generated have poor data quality. A study by LeadGen done in the last decade showed that running a lead generation program without properly cleansed data wastes on average 27.3% of a salesperson’s time.
- Invest your time and efforts in automated systems that de-duplicate, validate, verify, enrich and append your data.
- Technology empowered systems sift through large volumes of data using algorithms to detect irregularities and bad data.
- Regular data de-duplication, validation, verification and enrichment needs to be done as even good data goes bad.
8. Using authentic external data sources to append data
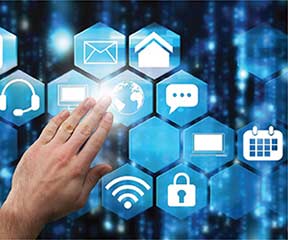
The rate at which data decays could range between 30% to 70% per year based on the countries, industries or businesses. New offices open, businesses change, takeovers and mergers etc. It is important to fill data gaps by cleansing and enhancing data value. Empty fields or incorrect data needs to be verified and appended by adding accurate and relevant information.
- Keep enriching datasets on a regular basis.
- Sync your clean data across systems.
- Use technology partners for quick and consistent data enrichment.
- Use authentic and reliable sources to append your data.
Clean data is the future of B2B
The future of B2B data management is all about hyper automation. With the growth of AI and ML tools, the focus of data cleansing has also changed. The aim now is to develop and deploy robust data cleansing processes that can prevent questionable data from entering the system whereas cleansing and validation are a continuous process.
Consult B2B data cleansing companies, invest in automation and technology-enabled tools to get the perfectly reliable data. Hiring a B2B data cleansing company with excellent technological infrastructure and reputation to assist you in all data cleaning requirements will prove to be a smart move.
Conclusion
Two things ring true when dealing with the complexity of handling real-time data today. The first is that real-time data aggregation is full of challenges, and the second is that there are enough advanced tools in the market that can overcome these challenges when used by B2B data cleansing experts.
There is virtually an arms race in managing B2B databases with pressure to acquire as much data as possible; but disorganised, inaccurate and unstructured data will lead to data accidents and generate false leads. Data quality needs to be optimized and properly validated and structured with contextual relevance, or big data will overwhelm and remain ineffective. Technology solutions and data experts provide the way out.
Want to get rid of bad data and manage unwanted outliers?
Hire our experts for advanced B2B data cleansing.
Get started today »