Data cleansing solutions leveraging on advanced data standardization & validation technologies can enrich your business-critical data for improved decision-making, accurate customer profiling and enhanced bottom lines.
Contents
In today’s data-driven world, businesses rely heavily on accurate and reliable information to make informed decisions and maintain a competitive edge. However, bad data can lead to increased costs, inefficiencies, and poor decision-making.
In this blog, we will explore the concept of data cleansing, its importance in addressing the prevalence of bad data, and its role in cost reduction for businesses.
Understanding the cost of bad data
Bad data refers to information that is inaccurate, incomplete, inconsistent, or outdated. It can take various forms, such as duplicate records, missing values, incorrect data entries, and inconsistent formatting.
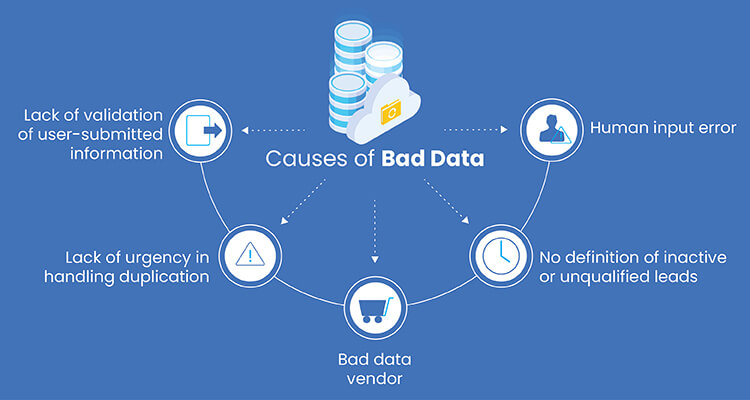
Gartner says poor quality data is expensive, costing businesses an average of $9.7 million per year and posing a massive problem for them
The prevalence of bad data is a significant concern for businesses, as it can lead to several negative consequences, including:
- Increased operational expenses due to time and resources spent on correcting errors
- Poor decision-making based on inaccurate information
- Loss of customer trust and loyalty due to inconsistent or incorrect data
- Regulatory fines and penalties for non-compliance with data quality standards
Bad data costs businesses an average of $15 million annually, according to Gartner’s Data Quality Market Survey. Given the potential impact of bad data on a business’s bottom line, it is crucial to address this issue through effective data cleansing practices.
In 2018, a fat-finger error occurred at Samsung. A securities South Korean employee mistook South Korea’s currency for shares, paying out 1000 Samsung Securities shares to employees instead of 1,000 won per share in dividends. In the end, this single human error cost $300 million to Samsung.
Similarly, an overcalculation of its commission cut caused Uber drivers to be underpaid. This incorrect formula circulating in its circulation cost tens of millions of dollars to Uber. They had to repay about $900 per driver.
Learn how to combat these costs with effective data cleansing.
Contact our data cleaning experts »Importance of data cleansing
Data cleansing, also known as data cleaning or data scrubbing, is the process of identifying and correcting or removing errors, inconsistencies, and inaccuracies in datasets. This practice involves various steps, including:
- Data auditing: Assessing the current state of data quality and identifying issues
- Data cleaning: Correcting or removing errors and inconsistencies in the data
- Data validation: Ensuring that cleaned data meets predefined quality standards
- Data monitoring: Continuously tracking data quality to maintain high standards
By implementing data cleansing, businesses can ensure that their data is accurate, consistent, and up to date, leading to better decision-making and operational efficiency.
Role of data cleansing in cost reduction
Data cleansing plays a vital role in reducing costs for businesses by:
- Eliminating duplicate records: Duplicate records can lead to increased storage and processing expenses. Data cleansing helps identify and remove these duplicates, reducing costs and streamlining operations.
- Improving data accuracy and consistency: Accurate and consistent data is essential for efficient business operations and decision-making. Data cleansing ensures that businesses have access to reliable information, leading to cost savings and improved performance.
- Reducing the risk of regulatory fines and penalties: Non-compliance with data quality standards results in substantial fines and penalties. Data cleansing helps businesses maintain compliance and avoid these costly consequences.
Implementing data cleansing to lower costs
Overview of data cleansing tools and services
There are various data cleansing tools available in the market, each offering unique features and capabilities to help businesses maintain high-quality data. Some popular data cleansing tools include:
- OpenRefine: An open-source tool for cleaning and transforming data, OpenRefine offers features such as data clustering, faceting, and filtering to help identify and correct data issues.
- DataWrangler: A web-based tool for cleaning and reshaping data, DataWrangler provides an intuitive interface for data manipulation and transformation, making it easy for users to address data quality issues.
- Trifacta: A data preparation platform that includes data cleansing capabilities, Trifacta offers advanced features such as data profiling, pattern recognition, and machine learning-assisted transformations to improve data quality.
Though these tools can help businesses save costs, they don’t help to meet customized requirements that your business might have. Outsourcing your data cleansing process to experienced service providers will help in the long run to reduce manual effort and ensure data quality.
Steps to integrate data cleansing into your business
To integrate data cleansing into your business, follow these steps:
- Assess your current data quality: Evaluate the state of your data quality and identify areas for improvement, such as duplicate records, missing values, incorrect data entries, and inconsistent formatting.
- Choose a data cleansing tool or service provider: Select a data cleansing tool or service provider that meets your needs and budget, considering factors such as ease of use, scalability, and integration with your existing systems.
- Develop a data cleansing strategy: Establish a data cleansing strategy that outlines your data quality goals, processes, and standards, as well as the roles and responsibilities of team members involved in data cleansing.
- Implement the data cleansing tool or services: Integrate the chosen data cleansing method into your business processes and systems, ensuring seamless data flow and efficient data management.
- Monitor and maintain data quality: Continuously track data quality and address any emerging data quality issues to maintain high standards and ensure the effectiveness of your data cleansing efforts.
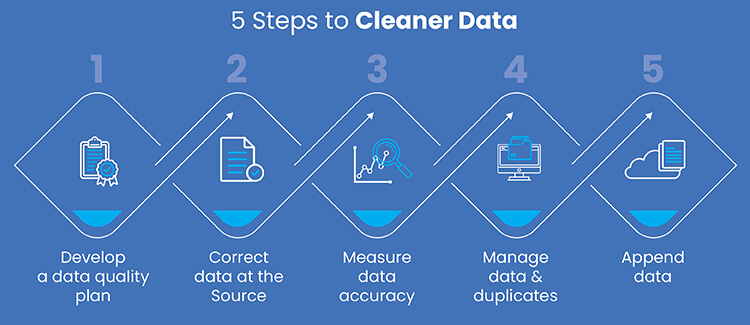
Role of training staff in data cleansing to further cost reduction
Training staff in data cleansing techniques and best practices can further reduce costs by:
- Ensuring data quality awareness: Educating employees on the importance of data quality and the consequences of bad data helps create a culture of data quality awareness and adherence to best practices.
- Reducing reliance on internal teams: Training staff in data cleansing techniques can build up on your costs, time and efforts. The need for external consultants or services is crucial to manage data quality, resulting in cost savings.
- Empowering employees to address data quality issues: Equipping employees with the skills and knowledge to identify and address data quality issues proactively helps maintain high-quality data and reduces the costs associated with correcting errors and inconsistencies.
In addition to direct cost savings, data cleansing also offers indirect cost savings for businesses:
Better decision-making: Accurate and reliable data enables businesses to make better-informed decisions, leading to cost savings through optimized strategies and reduced risk of costly mistakes.
Enhanced customer relationship management: Consistent and up-to-date customer information allows businesses to better understand and serve their customers, leading to increased customer satisfaction, loyalty, and reduced customer churn costs.
Improved operational efficiency: High-quality data streamlines business processes and improves productivity, resulting in cost savings through increased efficiency and reduced waste.
By integrating data cleansing into your business and training staff in data cleansing techniques, you can directly and indirectly reduce costs, improve decision-making, and maintain a competitive edge in the market. Investing in data cleansing is not only a smart business decision but also a necessary one.
Benefits of data cleansing
Cost savings from increased operational efficiency
- Streamlining data management processes: By identifying and correcting errors, inconsistencies, and inaccuracies in datasets, data cleansing streamlines data management processes, reducing the time and resources spent on manual data cleaning tasks.
- Reducing storage and processing costs: Eliminating duplicate records and maintaining accurate data can help reduce storage and processing costs, as businesses only need to store and process relevant, high-quality data.
- Improving productivity: High-quality data enables employees to work more efficiently, as they can trust the information they are using and spend less time addressing data quality issues.
Cost reduction from improved decision-making
- Enabling better-informed decisions: Clean, high-quality data provides businesses with the information they need to make better-informed decisions, leading to optimized strategies and reduced risk of costly mistakes.
- Facilitating data-driven insights: Accurate data allows businesses to generate valuable insights through data analysis, enabling them to identify opportunities for cost savings and revenue growth.
Cost benefits from enhanced customer relationship management
- Maintaining consistent and up-to-date customer information: Data cleansing ensures that customer information is accurate, consistent, and up to date, allowing businesses to better understand and serve their customers.
- Reducing customer churn: High-quality customer data enables businesses to deliver personalized and relevant experiences, leading to increased customer satisfaction, loyalty, and reduced customer churn costs.
Cost avoidance from better regulatory compliance
- Maintaining compliance with data protection regulations: Data cleansing helps businesses ensure that their customer data is accurate and up-to-date, reducing the risk of non-compliance with data protection regulations and avoiding potential fines and penalties.
- Avoiding costly audits and investigations: By maintaining high-quality data, businesses can reduce the likelihood of costly audits and investigations related to data quality issues.
With clean data, earn clear profits.
Get a FREE Quote »The future of data cleansing and cost control
As technology continues to evolve, we can expect several advancements in data cleansing technologies, including:
- Integration of artificial intelligence (AI) and machine learning: AI and machine learning algorithms can be used to automate the data cleansing process, identify patterns and anomalies in data, and make intelligent recommendations for data corrections and improvements.
- Automated data cleansing: AI and machine learning can automate the data cleansing process, reducing manual effort and associated labor costs.
- Improved accuracy and efficiency: AI and machine learning algorithms can identify and address data quality issues more accurately and efficiently than manual processes, leading to cost savings through reduced errors and improved data quality.
By leveraging AI and machine learning in data cleansing, businesses can unlock new cost-saving opportunities and further enhance their overall efficiency.
Conclusion
In conclusion, the future of data cleansing and cost control is promising, with upcoming advancements in data cleansing technologies and the integration of AI and machine learning offering new opportunities for cost savings and business efficiency.
By embracing these innovations and continuing to prioritize data quality, businesses can maintain a competitive edge in the market and drive growth.
It’s time to discover the real cost of dirty data and combat it.
Start a Conversation »