For real estate data aggregators, outsourcing pays rich dividends and remains central to their growth strategy, flexibility, scalability, resilience, and sustainability.
Comprehensive, current, and accurate property databases are indispensable for real estate data aggregators. Database quality defines their credibility and market acceptance. Ensuring high levels of data hygiene while harvesting data across channels on an ongoing basis, consumes much time and resource. On top of this, they need to manage operational issues like work volume fluctuations and access to right resources.
Outsourcing real estate support services is thus a logical choice for data aggregators. This is because established outsourcing companies add the operational scalability, skilled resources and ready infrastructure needed to overcome data aggregation challenges.
Property data not reported for 409 U.S. counties (13% of the country) like the Upper Peninsula of Michigan, northern Alaska, and southeast Utah resulted in enormous gaps in the property database of a leading real estate aggregator.
Source: NewAmerica.orgThe processes involved in collecting, cleansing, validating, and verifying data require significant investments in technological and human resources. Taking a constricted approach here leads to precarious business outcomes.
Top 5 challenges faced by real estate data aggregators
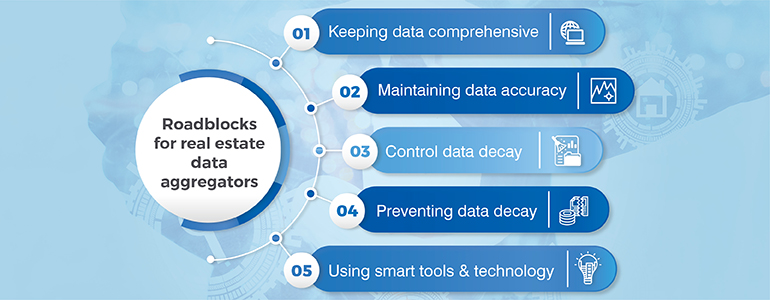
Property data-related tasks have increased exponentially with the advent of big data and omni-channel data accessibility. Deeper detection of pattents and trends in real estate is now possible. And data aggregators cannot afford to ignore client requirements for non-traditional data.
- Keeping data comprehensive
Omni-channel data capture is the foremost challenge for real estate data aggregators. With privacy law compliance on third-party cookies and consumers rightly conscious about their personal data the gathering of zero, first-party, second-party, and third-party data has become extremely difficult.
- Maintaining data accuracy
Inaccurate and incomplete data poses a typical challenge for data aggregators. Often, scrutiny of public records or upating of data lacks diligence. And credibility suffers when clients receive data that is outdated or lacks authenticity. To overcome this, aggregators need to constantly check dynamic public records, including:
- Parcel dataset – neighborhood
- Property transaction database
- Property tax database
- Property listing database
It is difficult to ensure that data extracted from readily available and obscure public sources is always transformed into a structured and easily accessible format. Managing, validating, cleansing, and normalizing data from multiple structured and unstructured sources is challenging when up against deadlines.
- Keeping dirty data at bay
Data aggregators use real-time property information as a force multiplier to arm their clients with deep property and customer intelligence. But it has an Achilles heel: dirty data. Dirty data manifests in various forms like duplicate data, inaccuracies, and other errors. Inaccurate and stagnant data are not reliable enough to show if a property title is free of any claims, liens, or other issues, and the workload inevitably increases.
- Preventing data decay
Keeping dynamic data attributes updated, refreshed and comprehensive poses a challenge for real estate data aggregators. These include vital information like property titles, mortgage, tax, appraisals, etc. Selling inaccurate, outdated, or incomplete property information to clients can jeopardize a real estate data company’s brand credibility and revenue streams.
- Using smart tools to leverage data attributes
Inability to gain and index property data points and keep them refreshed is an arduous challenge for aggregators. The lack of smart tooling capabilities makes it even more difficult. And there’s no single tool for all tasks. It is a complex task to identify, mix/match, and compile attributes like property data, mortgage and homeowner information, and real estate document images.
Besides regular data, like owner identification and pricing, and transactions, there is now a need for many more types of data in real estate. These may include plot shape and geospatial data, the proximity of combustible vegetation, or even social media ratings of nearby facilities. Investors, insurers, builders, and other stakeholders in the real estate sector can now put such data to good use with the help of new tools.
Outsource your real estate tasks and grow efficiently.
Tell us how we can help with your data aggregation
Get started today »Top 5 tasks real estate data aggregators should outsource
Real estate data aggregators collect property-related details from multiple sources, validate the information and make it available to MLS sites, insurers, agents, contractors and other stakeholders in the industry. Hence, they outsource structured tasks and focus in-house resources on growth. The tasks they outsource to achieve higher efficiencies include:
1. Property data acquisition
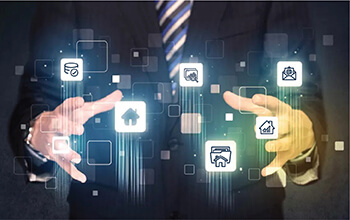
Much of the property data is not readily available on digital sources and still lies in paper deeds, leases, licenses, agreements, and other documents.
Outsourced data acquisition of 110 million property records helped a leading real estate portal serve 200 million online customers browse home and apartment listings and shop for mortgages conveniently.
Common tasks in property data acquisition include:
- Detecting authentic property data sources, both global and local.
- Real-time raw data harvesting from public records and other sources like newspapers.
- Getting property tax data from state, county, and sub-taxing websites.
- Collecting real estate data from obscure and non-traditional sources.
- Creating an ongoing property data acquisition process/cycle.
- Building a comprehensive real estate data repository.
2. Standardization of property data

A property consultant and MLS site from the UK enhanced its brand credibility and customer experience by outsourcing real estate data tasks. A company proficient in leveraging Google Maps and Google Street View collected and standardized unstructured property data from many brochures and various agencies.
In the case of multiple listing services, where data is assumed to be structured, there are hundreds of sources with their own ways of structuring data and there’s no standardization. Even where such data is available in ready digital format, integrating listings from multiple sources becomes complicated and requires time.
Common tasks in the standardization of property data include:
- Data normalization
- Address normalization
- Normalization of geographic data (state, county, city, country, etc.)
- Assigning Zip codes and geographic indicators wherever possible
3. Rule-based property data validation and verification
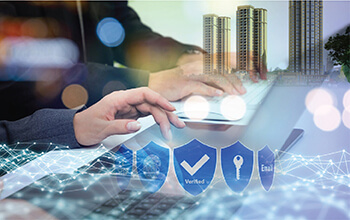
Validation of real estate property data is extremely important from the angle of accuracy, compliance and data hygiene for analytical utility. Both manual and rule-based property data validation and verification are used for this purpose.
Outsourcing validation and verification of over 10 million records helped a US-based publisher of real estate periodicals increase circulation and enhance credibility by providing accurate insights and data to subscribers.
Common data validation tasks include:
- Postal address validation
- Demographic validation
- GIS data validation
- Reverse phone lookup
- IP address validation
- Property data validation
- Agent data validation
- Mobile and email validation
4. Property data enrichment
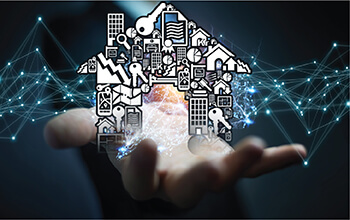
Both traditional and non-traditional property data requirements have increased the workload of data aggregators. Property datasets may now require enrichment with new data such as crime statistics, building footprint, weather patterns, traffic statistics, etc.
Common data enrichment tasks include:
- Sociographic, demographic, and other relevant data in public records.
- Residential, commercial, financial, geospatial, and rental information.
- Filling cross-property fields with data from multiple MLS listings.
- Addition of images and other attachments.
- Identification of missing fields, the addition of relevant values, and reaching a zero missing-value status.
5. Continuous listing updates

The threat of data decay looms over the operations of every real estate data aggregator. So, they put automated systems in place, creating continuous data cycles to update, validate, and enrich property listings.
Real estate outsourcing services set up an autonomous data process to search and collect property information from 1,350+ multiple listing services. It helped the New York-based real estate portal to provide an easy-to-access database to clients across counties.
Thankfully, most current real estate data is public and digitally available to aggregators who have automated data monitoring capabilities. They can set up reliable systems to detect any information change or outlier as soon as they appear on the digital horizon.
Common tasks associated with a continuous update of listings include:
- Ongoing and accurate property listing management
- Leveraging macros and business rules to update the database with relevant transactional values in real-time
- Capturing and updating pricing fluctuations/asset valuation
- Automated tracking and monitoring of changes in existing data
- Removal of redundant and obsolete data from the database on the go
Deploy technology advancements to grow profitably
Artificial intelligence (AI) and Machine learning (ML) models are revolutionizing the real estate industry. The opportunities that AI comes with are already assisting listing sites to understand and dissect data for securing sales. ML algorithms help realtors to assess and note down key features of properties in a particular area that are likely to affect the selling price.
They can program ML models to accumulate data on interior features inside a house, categorize them, and then correlate them to the value of the property. Similarly, natural language processing (NLP) can be leveraged to determine ideal clients for a specific property by matching people with their desired property through high-value touchpoints. Property listing sites can use expert help to deploy computer vision technology to plan and organize listing images to improve user experience.
All these require skilled resources which are difficult to manage in-house. These tasks are time-consuming and require a high level of attention and diligence, hence better outsourced to property data management experts.
Conclusion
As all modern industry sectors are adopting data-driven mindsets, real estate developers or investors are not the only people looking for real estate data. Insurers, builders, engineers, architects, etc., in the real estate arena now use data-driven solutions that require a wider and varied range of contextual data on top of traditional information.
To stay competitive, real estate data aggregators thus have to weave in huge amounts of available and disparate data and remain always in need of quality support. Therefore, real estate data aggregators should outsource demanding tasks to real estate support services. It helps them to ensure business growth by freeing up core resources and using them wherever they are needed most.
Want to increase efficiency and boost profitability?
Talk to our property data management experts.
Get started today »